“Explainability” for AI and ML in Data Privacy
• read
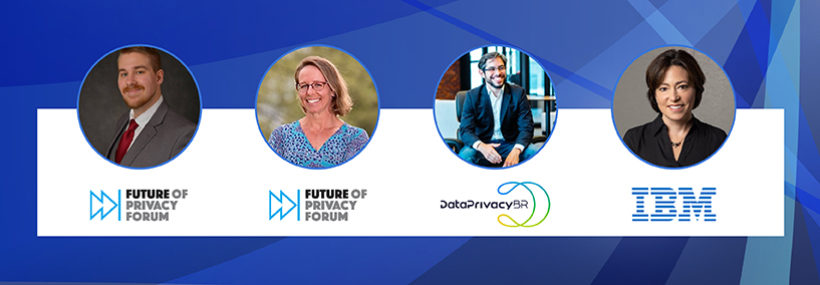
How to Implement “Explainability” in Emerging Global AI/ML Regulations
Explainability is defined in various ways but is at its core about the translation of technical concepts and decision outputs into intelligible, comprehensible formats suitable for evaluation.
—Fjeld, et al., 2020 [1]
Artificial Intelligence (AI) and Machine Learning (ML) are opaque: even for the scientist creating and working with these systems. As award-winning AI researcher and IBM Watson team founder, David Ferruci, opined, AI may be building intelligence that “may have little do with how to engage with, and reason about, the world. And critically, “How useful is that model when we need to probe it and understand it?”[2]
The need to understand automated decision-making is increasingly a concern in the privacy policy environs. If scientists struggle with it, how is it made “intelligible” to the average consumer? Furthermore, “explainability is not exactly the same thing as transparency,” informs Future Of Privacy Forum’s Lee Matheson. “And it is a concept that comes up a lot, specifically in connection with AI automated decision making and machine learning (ML) technologies.”
To discuss the challenge of AI explainability in the context of data subject rights, Matheson moderated a panel of privacy and AI experts at the Fall Spokes Privacy Technology Conference. Joining him, were his FOPF colleague Dr. Sara R. Jordan, Sr Researcher, Artificial Intelligence & Ethics; Christina Montgomery, Vice President & CPO, IBM; and Renato Leite Monteiro, Co-Founder of DataPrivacy Brazil, who was closely involved in the drafting of Brazil’s General Data Protection Law You can view the conversation, “Explaining AI: How to Implement “Explainability” in Emerging Global AI/ML Regulations,” here.
Explaining Explainability
I key in two words that they use to support the definition: intelligibility and comprehensibility. And making something intelligible really depends on the target audience that you’re trying to make it intelligent for. And then comprehensibility depends upon the audience’s ability to take that information…on board and to do something with it.
—Dr. Sara R. Jordan, Future Of Privacy Forum
“My idea of explainability is much more than providing an explanation of the technical concepts,” says Monteiro. “It is allowing data subjects to use the information provided to them to possibly challenge automated decisions to guarantee their fundamental rights.” He sees four limitations to this:
- The technical;
- Legal limitations involving commercial secrets and IP;
- How to translate technical concepts for the data subject; and,
- Institutional. Who is going to enforce the explanation?
Montgomery views explainability “as information related to the particular decision and why the decision was made….a way to enhance transparency about what went into the system, how it was trained, the data that was used, and how it’s deployed related to the decision itself.”
“[But] being able to provide meaningful explanations to an individual user or to the data scientist that’s developing the AI application, those are very different things.”
And context matters, she says. Some applications are very high stakes and the level of granularity required would be very different from what you’d expect in a low-stakes context like retail.
Explainability and existing privacy regulation
“When you look at regulation like the GDPR it’s addressing personal information in a technologically neutral way,” notes Montgomery. “It’s also covered from the perspective of the main underlying principles of the GDPR like the concepts of lawfulness, fairness, and transparency.
“The GDPR, with respect to AI specifically, is only in the context of an automated decision without human oversight.” As such, there’s a very limited subset of AI systems, to which the GDPR applies.”
The existence of the right to explanation in the Brazilian General Data Protection Law (GDPL) is the core of Monteiro’s Ph.D. research.
“I go to Articles 13, 14, and 15, and recital 71 of GDPR and so forth,” says Monteiro, “where it is not enshrined in the regulation. If we are to advocate there is a right to explanation in the Brazilian legislation, we need to derive that from other sources.”
I think we can say that there is a right to explanation under Brazilian law. But there’s one particular concept that is different from GDPR and U.S. Personal data can be anything that allows for singling out the person, but there is one particular article under the Brazilian law that says even anonymized or non-personal data, if it is used for profiling purpose [e.g., demographic data], it will be deemed personal data.
—Renato Leite Monteiro, DataPrivacy Brazil
Simulate-ability, decompose-ability, and interpretation
Jordan notes that there really is really no “academic consensus yet in terms of what explainability is” and suggests as required reading the paper Explainable Artificial Intelligence (XAI) that provides a landscape of explainable AI.” [3]
“What I think is useful about [the paper] is that it does try to decompose the idea of explainable AI into at least three parts: simulatability, decomposability [and interpretability]:
- Simulatability is the ability of you to think as if you’re the computer– can you reimagine what it means to try to make this decision – if you were given infinite time, space, and the ability to compute this What would you come up with?
- Decomposability is the ability to break down what a system does. What happens to the data about you? How is it brought together? How is it turned into features? How is it brought into systems and manipulated to get to some sort of output?
- Interpretability of AI. Is it something that you can take on board and that you can do something with in your life?”
“If there’s one area in which I think there is emerging academic consensus”, says Jordan, “it’s that interpretability and explainability is audience-specific…”
Explicability in practice
At IBM “we’re focused on building the capabilities through something we call a governance into those into our general-purpose AI systems,” says Montgomery. One example offered is the ability of IBM products to automatically produce “fact sheets” across the development and deployment process that creates a log about data being used, the tests conducted, and outputs.
[Fact sheets are] reflective of the model development lifecycle and also capture things like bias and privacy measurements. The goal is ultimately to produce something that will be able to provide transparency…and will be tailored and context-specific.
Say it’s in the context of a loan application that helps support decisions… the loan officer would get a different explanation – a different fact sheet – than the data scientists who created it.
—Christina Montgomery, IBM
But it’s important to remember that “the AI ecosystem involves many players throughout the lifecycle and it’s very complex,” cautions Montgomery, “so you can’t look at this as a simple supplier environment as you would in a typical product.”
As a company, and leader in AI, IBM “thinks about guardrails all the time and whether they can be built in technically” as part of their AI governance model, she continues. “There are technical abilities to do that, but we don’t think about just the technical. It is thought about holistically.”
AI explainability for regulators
To talk to a regulator, you have to remember what their purpose is. We’re not talking to a general-purpose regulator. We are talking to regulators within particular verticals that have a particular remit [and require the AI to provide] a sufficient explanation for them to be able to fulfill their remit.
This addresses the challenge of explainability, within use contexts, but also the individual. If you look at the difference between what regulators need and what individual people need.
—Sara R. Jordan, Future Of Privacy Forum
And, as Jordan rightfully notes, there is sometimes a massive gulf between what regulators and the general public require in terms of explainability.
“This is not to say that there is an absolute divide, but rather, keeping in mind that they have different purposes. Individuals need to protect themselves, their families, their communities. And regulators need to protect all of those and within a particular vertical.
“So, it’s going to be very narrowly tailored. Trying to do something extraordinarily general on explainable AI – it’s theoretically necessary – but it may not be practically useful just yet.”
The black box problem
Echoing Ferruci’s concerns, Monteiro notes that “Some technologies will, by design, not be explainable. And that’s one of the things that we need to discuss if we want explainability-by-design embedded in it.
“This is useful for understanding the difference between transparency obligations (ex-ante) and explainability (ex-post) obligations.
“When we are talking about ex-ante obligations,” explains Monteiro, “we are talking about transparency, in order to say what data is used, what are the inputs creating output, and so forth. When you’re talking about ex-post obligations, we are talking about explanations and accountability measures.
Explainability – even on non-explainable systems – can work, not only for the data subject to challenge that particular decision, but also to provide the regulated enforcement authority enough comprehensibility of the system to check if it follows the compliance obligations.
One of the ways to do this is to provide the purpose and impact that particular automated system can have on people. Also, if you cannot properly explain the technical concepts of it, can you demonstrate the impact if you change some of the variables?
—Renato Leite Monteiro, DataPrivacy Brazil
Matheson raises an important point: “It’s one thing to provide a counterfactual explanation for a data subject to illustrate how a given system would produce outputs given certain inputs, but how does that work for someone who wants to exercise, for example, a right to challenge a particular decision? How do you insert a human into a process?”
Montgomery cautions that “there should always be a human in the loop,” and I think that’s how you, in part, get around this idea that maybe you won’t be able to fully explain the output of every decision in a sufficiently satisfactory way.” Particularly if the output is a recommendation.
Perhaps, but as Jordan highlights, “one of the key things to remember here is most of the systems are just not explainable as yet.”
Watch the entire SPOKES session here