Privacy Law Impacts to AI and High-Risk Processing
• read
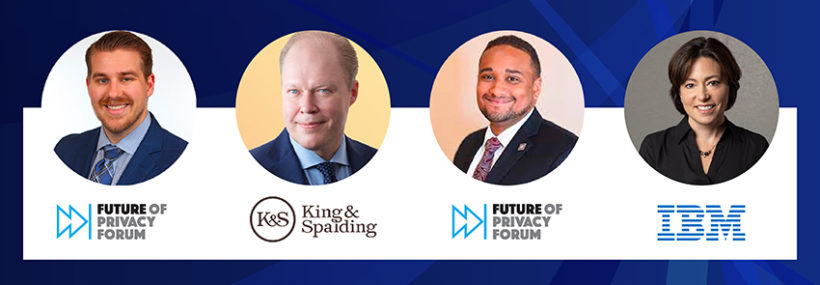
AI and High-Risk Processing
Day one of WireWheel’s Summer 2022 Spokes Data Privacy Technology Conference (held June 2-3) featured the discussion AI and High Risk Processing focusing on issues concerning regulation and development of privacy law concerning artificial intelligence (AI) and automated decision making.
Moderator Lee Matheson, Senior Counsel for Global Privacy at the Future of Privacy Forum was joined by several leading experts including his colleague Bertram Lee, Sr. Counsel on Data and AI Future of Privacy Forum. Notably, Lee very recently gave testimony before the House Commerce Committee on the newly introduced Proposal for American Federal Data Privacy Law.
Also joining was King & Spalding LLP Data Privacy & Security Partner Jarno Vanto and Christina Montgomery, Vice President, CPO, and Chair of the AI Ethics Board at IBM.
Montgomery also serves as a member of the Commission for Artificial Intelligence Competitiveness and Inclusiveness as part of the U.S. Chamber of Commerce and has recently been appointed to the United States Department of Commerce’s National Artificial Intelligence Advisory Committee.
The Artificial Intelligence (AI) regulatory state of play
In terms of how artificial intelligence is starting to get regulated we’re seeing the world fall into three different camps:
- The more prescriptive approach, adopted by the European Union, where you have a regulation on what types of AI cannot exist, what types of AI are high risk, and what types of AI are low risk.
- A self-regulatory environment coupled by government enforcement when the self-regulatory frameworks fail.
- The sectoral approach, currently prevalent in the United States with different government entities issuing rules and statements about AI in the sectors that they administer
One strategy companies are using to deal with the “sectoral approach” is looking at which regime to follow. “What I’m starting to see is that it’s just easier for companies to comply with the strictest regime.”
In some ways, the EU has emerged as a global regulator of artificial intelligence, at least with the initial steps they’ve taken. And unless companies are willing to build regional AI tools that comply with the local regulations (which would be enormously costly and difficult to manage) it would mean that standards around [prohibited] AI, or judgment calls around what constitutes “high risk,” would be adopted globally.
—Jarno Vanto, King & Spalding LLP
Of course, U.S. companies may view these EU efforts as mandates on U.S. companies, given the fact that most of these AI tools are currently being developed in the United States.
How to approach artificial intelligence (AI) governance?
Our approach – even in the absence of regulation – is to start with your values and your principles. I think that’s the only way to design a governance program around AI, because so much of it has to be values led.
—Christina Montgomery, IBM
“The core of internal governance over AI regardless of the regulatory environment is the ethics and principles that govern development,” offers Matheson. What the law says matters of course, but certainly there are broader ethical considerations “like what do we want the company to stand for?”
These considerations are not solely tied “to how algorithms make use of data, but more broadly with use of data generally,” opines Vanto. “When developing these tools, the first thing companies should keep an eye out for is the purpose for which the tools will be used.”
Many companies have implemented data use-case ethics boards and similar bodies to contemplate this and will say no to the potential monetary gains if they view the use-case as unethical or inconsistent with their approach to use the personal information.
—Jarno Vanto, King & Spalding LLP
“With AI, it is the very same assessment, continues Vanto. “Is the purpose consistent with the values of your company?”
Civil rights advocacy and artificial intelligence (AI)
You can’t just rely on civil society groups to do the work that companies should be doing themselves. Ideally you would want civil rights feedback to come from inside the company…so that when these products and services are presented to advocacy organizations, the thoughts and considerations of those communities have already been thought through.
—Bertram Lee, Future of Privacy Forum
“From a policy perspective,” continues Lee, “one thing that might be helpful to think about with respect to the civil and human rights community is that Civil Rights law has prohibited discrimination in a variety of contexts, and in a variety of ways for the better part of 60 years.
“That context is important because when I hear from companies that the law isn’t clear [the question then becomes] how are you compliant in spirit? What is your best effort…with respect to non-discrimination?”
There is coming regulation on privacy. No doubt there is going to be some form of algorithmic mandate or accountability from Colorado or California, maybe even Virginia…The algorithmic biases issue is on its way.
For everyone involved, it makes the most sense to think about how to test for nondiscrimination. How your data sets are discriminatory, and how you’re fighting against that actively. What are the ways in which this AI could be used that could be discriminatory?
—Bertram Lee, Future of Privacy Forum
“All should be asked and answered before even thinking about deployment and there should be a clear reasoning behind it,” assets Lee. “The recent settlement with HUD is an example and folks are slowly waking up to their liabilities in this space.”
Managing artificial intelligence (AI) principals at scale
“How do we affect real transparency for a complex algorithmic system?” asks Matheson. “How do we regulate data quality for training data sets that have billions of data points – especially on the scale of IBM?”
“We have to make it easy for our clients,” says Montgomery. “That the tools we deploy are giving them the capabilities they need to be confident that the uses they’re putting AI to are fair, transparent, explainable, and nondiscriminatory.
“The IBM Ethics Board and the Project Office that supports it is within my purview of responsibility. We designed our program to be very much top-down bottom-up because we wanted the tone from the top…helping set the risk for the company, instill its importance, and hold us accountable…But importantly, also ensure that multiple voices are heard. That we’re incorporating the voices of marginalized communities as well.”
Montgomery further notes that IBM has approximately 250,000 employees globally and has created a network of multidisciplinary “focal points” throughout the company that comprises both formal roles and an advocacy network of volunteers to support this effort. The result is a culture of trustworthiness.
The how is where it becomes really tricky. It’s one thing to have principles. It’s one thing to have a governance process which is really central to holding ourselves accountable and helping to operationalize those principles. But we have to tell people how.
—Christina Montgomery, IBM
IBM has a living document called Tech Ethics by Design explains Montgomery. It walks designers and developers through the questions they should be asking and through the tools they should be using to test for bias. And it gives them data sheets to document the data being used throughout every stage of the lifecycle.
But IBM doesn’t go it alone says Montgomery. IBM also collaborates with external organizations like the open-source community and is currently funding a lab at the University of Notre Dame.
Will regulation help with the issue of artificial intelligence (AI) bias?
“We often see – whether it’s a prescriptive regulation or voluntary self-regulatory framework, or even just a statement of principles – people get lost in the weeds of how to do the compliance,” says Matheson.
“I would love to see a co-regulatory approach, says Montgomery, “and IBM has been calling for precision regulation of artificial intelligence to regulate the high-risk uses. Not regulate the technology itself. We’re supportive of guidance, frameworks, and regulation in this space, but it’s important to have that regulation be informed by what businesses are seeing and balancing innovation and risk.”
“I agree,” says Vanto, “actual business practices should be factored in so regulatory work doesn’t happen in a vacuum. But it’s interesting, if you’re looking for example, the list of the high-risk and prohibited systems, they’re value-based judgments.” This shouldn’t be set in stone as there a use cases that we can’t even conceive of today, and “having that in regulation that takes years to change, may be challenging.”
Instead of setting in stone what constitutes a high-risk activity now – the prescriptive approach – we should have certain criteria based on which certain systems or use cases should be considered high risk or prohibited altogether…because there might be others down the line very quickly as these things develop.
—Jarno Vanto, King & Spalding LLP
Self-regulating artificial intelligence (AI)
“While I agree that we don’t want to necessarily stifle innovation – there are ways in which these technologies could use to benefit all of society – we have to understand that the data sets that underlie a lot of these systems are all based in discrimination,” contends Lee.
“If folks could self-regulate, we wouldn’t be having some of the same problems that we’re having right now, because there would have been someone who was in the room, saying let’s revaluate.”
As an example of internal evaluation working, Matheson notes that IBM chose not to offer API’s for facial recognition software which was publicly announced.
It comes back to the first point I made underpinning our governance framework are the values and the principles that we align ourselves to, beginning with data should augment not replace human decision making. It should be fair, transparent, explicable, privacy-preserving, secure and robust.
—Christina Montgomery, IBM
Montgomery notes that IBM had a number of proposals come forward during COVID-19 to, for example, use facial recognition for mask detection or fever detection for deployment in various airports or businesses. The concern was how guard rails could be put around the different technology types. The details of IBM’s decision making process were published by IBM in the report “Responsible Data Use During a Global Pandemic.”
“Ultimately, facial recognition (at least at the time) presented concerns regarding accuracy, fairness, and the potential for it to be used for mass surveillance or racial profiling. That coupled with the questions around the technology itself led IBM to the decision [not to deploy] facial recognition.
“We wanted to be very clear that we weren’t making different decisions, just because we were faced with this exigent circumstance. We were still relying on our governance process and still adhering to our values and our principles,” declares Montgomery.
This last point cannot be stressed enough. To jettison principals and values, even in exigent circumstances (the rallying cry of a long line of malefactors) renders the very concept of values and principals as nothing more than expediencies to be used or tossed as circumstances “require.” The very antithesis of “principals.”